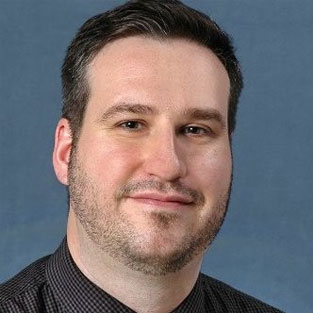
Brian Shappell, CBF, Director of Content Strategy
18th January, 2024
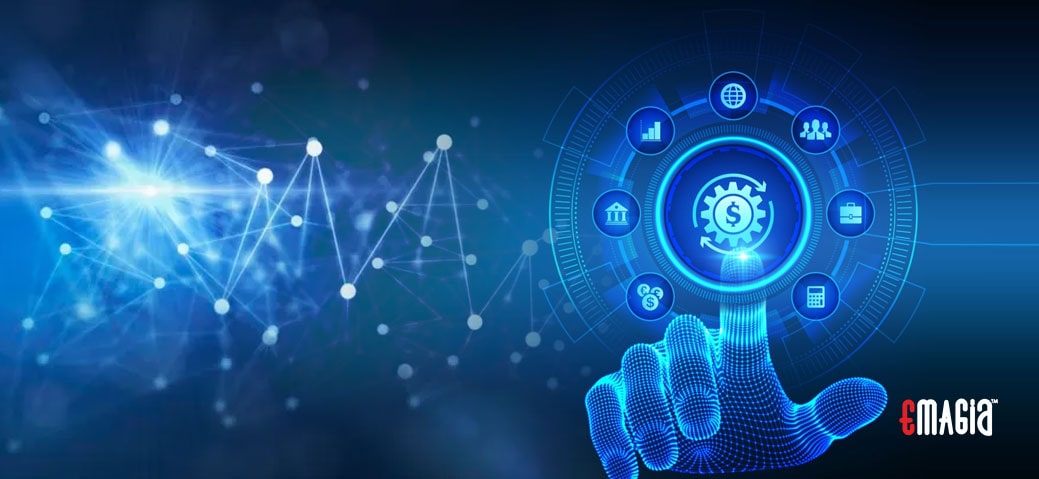
The ability to make informed and confident decisions quickly is imperative for the success of businesses, whether they are burgeoning startups or established, large enterprises. Financial planning and forecasting stand out as indispensable tools that empower CFOs and finance leaders to make strategic decisions. Traditionally, finance teams relied on spreadsheets or forecasting embedded ERP software for creating financial forecasts.
However, forecasting tools and ERPs demand extensive training and significant investments, rendering them inaccessible for many small and medium-sized businesses. Meanwhile, more accessible spreadsheets involve a time-consuming process requiring expertise in modeling techniques, among other things, with an inherent risk of errors.
The advent of Generative AI (GenAI) is poised to revolutionize finance functions, offering instant generation of customized and intricate financial forecasts. This allows for real-time revisions and iterations without relying on a robust in-house finance team.
“Generative AI can explain forecast and budget variances for financial planning and analysis teams to use in business reviews – It can also synthesize those trends and insights for executives and the board,” said Alexander Bant, a vice president in Gartner’s finance practice.
The Emerging Opportunities for Generative AI in Financial Forecasting
GenAI and financial forecasting processes have a sort of natural common ground, creating ample opportunity for inclusion of the emerging technology. It’s not by design, of course – after all, they were initially developed at very different times – but it is hard to ignore the possibilities.
Financial forecasting is the process of projecting the financial results of an organization by examining and basing on historical data, such as revenue, cash flow, expenses, or sales. This involves assumptions, as many unknown factors can impact future business performance. Financial forecasting empowers organizations in decision-making regarding human resources, budgeting, revenue outlook, and strategic planning.
Gen AI leverages machine learning from vast data sets, grants businesses the power to generate and update financial forecasts rapidly, efficiently, and accurately. It enables organizations of all sizes to make informed decisions using financial forecasts.
Increasingly, CFOs need to collaborate with upper management and FP&A teams to allocate investments for GenAI, incorporating AI-generated data and insights into the business forecast and plan. As the aforementioned Bant noted during a recent conference of CFOs and financial executives in London, C-suite and board members are increasingly enamored with GenAI business potential. Moreover, investors perpetually want new sources of growth and productivity – and they pay close attention to the improvement (or lack thereof) of margins.
“This is placing pressure on [finance] leaders to not get left behind in the AI race,” Bant warned.
To successfully adopt GenAI models, tools, and external solutions – like Emagia’s finance- and credit-centric GiaGPT – financial leadership teams must work collectively with other departmental leaders within the business to ensure proper adoption and address obstacles.
The following are key opportunities and/or advantages of GenAI in enabling businesses to make what we at Emagia like to call “faster, bolder” decisions:
- Real-time Modification and Adaptability: Financial plans and forecasts are increasingly dynamic, requiring swift modifications in response to market volatility, regulatory shifts, or internal developments. GenAI facilitates dynamic modification of financial forecasts, simplifying the process and allowing stakeholders from various departments to participate effectively.
- Risk and Scenario Analysis: GenAI simulates various scenarios to assess the impact of external factors on financial results. It enables businesses to identify potential risks, fostering a proactive approach to risk management for organizations of any size or stage.
- Informed Decision Making: GenAI enhances the accuracy and reliability of financial forecasting by utilizing large amounts of data. Real-time analysis of historical data and external data sources enables data-driven decision-making, eliminating cognitive biases and reducing subjective interpretations.
- Predictive and Variance Analysis: GenAI models provide insights into variations between forecasts and actual results, allowing quick identification of root causes and corrective measures. This empowers businesses to make sense of critical trends and patterns, facilitating data-driven financial decision-making.
Challenges of Generative AI in Finance
While opportunities for GenAI in finance are abundant, existing challenges require judicious addressing:
- Regulatory and Governance Framework: The absence of a regulatory framework for GenAI in finance raises concerns about fairness, accountability, and transparency, particularly in financial services.
- Security and Privacy: Security and privacy challenges arise from the sensitivity of financial data. Organizations must protect extensive volumes of sensitive data from compromise, as generative AI models, if compromised, could lead to significant consequences. This makes the vetting of external providers of AI-based products and services critically important.
- Interpretability: Complex GenAI algorithms may autonomously generate key financial decisions without human understanding, raising concerns about accuracy and transparency. Improving transparency in decision-making driven by generative models is essential for finance teams.
- Biased Decision: If unchecked, systematic errors in GenAI models can lead to biased decisions, sustaining stereotypes or creating wrong representations of different groups. Addressing biases in training data and model architecture is crucial.
- Data Accuracy: Earlier versions of GenAI tools lacked accuracy in output generation. Advanced features in models like GPT-4 are expected to address these challenges, enhancing accuracy in data generation. Meanwhile, other financial leaders have sought out more “closed-cloud” solutions.
- Barriers to Adoption: The steep learning curve associated with forecasting tools poses a bottleneck to the adoption of GenAI. Overcoming this learning curve with proper training and frank conversations to alleviate staff concerns is crucial to leveraging the technology effectively in planning and forecasting.
Concluding Note
CFOs and finance leaders must guide their teams in addressing the challenges and opportunities presented by the adoption of generative AI in finance. A KPMG survey found that 83% of respondents use GenAI for financial planning. As GenAI advances, the future of financial planning and forecasting looks promising, with improvements in accuracy, speed, and usability. However, organizations must take the time to ensure loose ends do not exist in areas like ensuring compliance with regulations, protecting sensitive data, and garnering buy-in from the various levels of staffers who will be using these newer AI-based often to drive business improvements.